Tyler Joiner, Binding P&C Director, shares 10 key tips for successfully leveraging data in the insurance industry, from balancing analytics with real-world insights to improving decision-making.
Evaluating and underwriting risk involves a balance of art and science. I know this firsthand: three years ago, I moved into an insurance product leadership role after 15 years in data science and analytics. Whether you're a seasoned data scientist, looking to leverage data to enhance portfolio management, or just curious about the “magic” of analytics, I’m excited to share my top insights and lessons learned from two sides of the insurance industry:
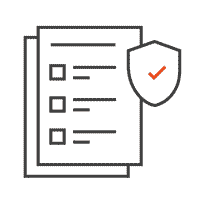
#1 You can’t use data that hasn’t been collected. This one may seem obvious, but it’s true and so important. Your best asset in predictive modeling and analytics is data, but you can’t use what you don’t have! It’s never too early to start collecting data that you wish you already had, knowing you will likely need it again.
Collecting that data using basic data integrity best practices—capturing it accurately, consistently and comprehensively—is key. Bonus points if you work with your organization’s Enterprise Data team to make your data officially “official."
#2 Data doesn’t lie, until it does… Data is the foundation for all things analytics, but you have to know its limits. If you notice a completely unexpected trend, dig into it. Either you’ll learn something that can enhance decision making or you’ll discover a confounding variable.
For example, we may see a higher bind ratio for business quoted on Wednesdays. Taking that at face value and instructing underwriters to only quote on one day of the week is the wrong conclusion. What if, instead, we go deeper and find that Wednesdays are the most common day for underwriters to be on the road, quoting from their trading partners’ desks? Day of the week isn’t driving the high bind activity, meeting our trading partners’ needs is.
#3 There’s no way to speed up time to gain data insights, although when it comes to experimentation, I wish there were. For time-based experiments where months needs to pass in order to determine the outcome (i.e., does one type of account bind more often than another), there’s unfortunately no substitute for waiting it out.
#4 Don’t substitute data for on-the-ground knowledge, specifically, leveraging relationships with your trading partners. Open communication between underwriters and brokers is key to keeping pulse on market shifts and how others are responding. In fact, the information received from trading partners is effectively the most updated data we could ask for. If we could capture it as quickly as our partners notice it, we would!
#5 Statistical significance shouldn’t replace common sense. In data science, we rely a lot on statistical significance through things like p-values (probability values). Don’t let an arbitrary cut-off determine how decisions are made.
Instead, make sure to take what data suggests and blend it with your own knowledge of the world. For example, posting a “No Trespassing” sign outside a premise may not be a statistically significant driver of loss reduction. Posting one also doesn’t cost very much money and is easy to do, so requiring a sign may be a no-brainer.
#6 The best model ever built is worthless if it isn’t used, whether due to mistrust or the inability to implement it. Sometimes, simple is better, especially if simplicity is what leads to buy-in, which in turn opens the door to more complicated model builds. On that note:
#7 Change management is often more difficult than the model building itself, but it’s critical to moving an organization forward from a data and analytics perspective. Do the hard work to get leaders (at all levels) to buy in. It'll pay off.
#8 Keep in mind factors that may not be reflected in data, and therefore can’t be modeled, such as institutional knowledge, technical training and expertise. For these things, we must rely on our underwriters. Loss experience on a portfolio can be as much a function of coverage and underwriting strategy as of specific risk attributes. There’s no replacing subject matter expertise, nor the “art” of underwriting.
#9 Just because something wasn’t possible a year ago, doesn’t mean it isn’t possible today. Technology is changing rapidly, and it’s often worth circling back to a good idea that wasn’t feasible in the past.
Large language models (LLMs) are one area where technology has advanced exponentially in recent months with no signs of slowing. Chatbots, that even a year ago seemed clunky and awkward, are now delivering answers that sound like they’re coming from a real person.
This is just one example. The big take-away is this: if there is value in a certain data insight or technological solution, don’t be afraid to try something again. Sometimes, the existing tools were the limitation, rather than the idea being a bad one.
#10 Have fun!
Use your imagination and don’t be afraid to fail. Changing the status quo means thinking outside of the box and testing out new ideas. They won’t all work out, but the ones that do, can be needle movers!